Scope and Background
Reinforcement learning (RL) is an active field of research that deals with the problem of (single or multiple agents') sequential decision-making in unknown and possibly partially observable domains, whose dynamics may be deterministic, stochastic or adversarial. In the last few years, we have seen a growing interest in RL from both research communities and industries, and recent developments in exploration-exploitation, credit assignment, policy search, model learning, transfer/hierarchical/interactive learning, online/multi-task learning, planning, and representation learning are making RL more and more appealing to real-world applications, with promising results in challenging domains such as recommendation systems, computer games, financial marketing, intelligent transportation systems, healthcare and robotic control. After great sucesses in the past four AWRL workshops held in Hamilton, New Zealand (2016), Seoul, Korea (2017), Beijing, China (2018, 2019), the 5th AWRL workshop focuses on both theoretical models, frameworks, algorithms and analysis of RL, as well as its practical applications in various real-life domains. The half-day workshop consists of sessions devoted to invited talks on specific topics on RL and presentations on publications in top conferences such as AAMAS, AAAI, IJCAI, KDD, ICML, NeurIPS. The ultimate goal is to bring together diverse viewpoints in the RL area in an attempt to consolidate the common ground, identify new research directions, and promote the rapid advance of RL research community.
Invited Speakers
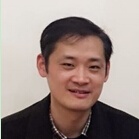
Xin Xu
National University of Defense Technology
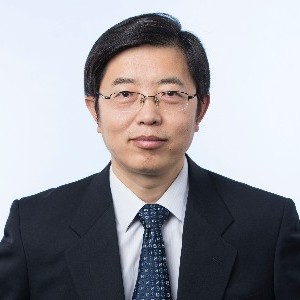
Dongbin Zhao
Chinese Academy of Sciences
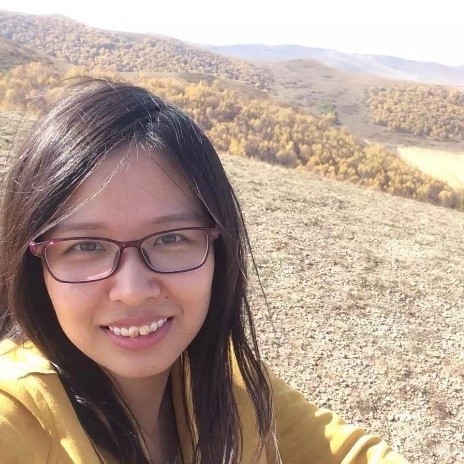
Li Zhao
Microsoft Research Asia
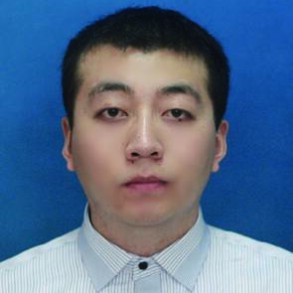
Xian Guo
Nankai University
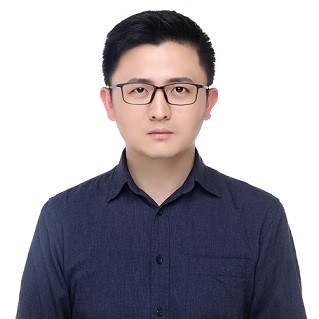
Junge Zhang
Chinese Academy of Sciences
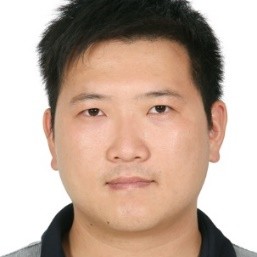
Feng Wu
University of Science and Technology of China
Overall Program
9:00-9:40 (GMT+8) | Reinforcement Learning for Optimized Decision-making and Control of Intelligent Robots | Xin Xu | National University of Defense Technology |
9:40-10:20 (GMT+8) | Artificial intelligence methods in real-time fighting game | Dongbin Zhao | Chinese Academy of Sciences |
10:20-10:50 (GMT+8) | Reward Decomposition: Discover and Leverage Decomposable Structure in Deep Reinforcement Learning | Li Zhao | Microsoft Research Asia |
10:50-11:20 (GMT+8) | Path integral reinforcement learning and its application in control system | Xian Guo | Nankai University |
11:20-11:50 (GMT+8) | Model-based Reinforcement Learning | Junge Zhang | Chinese Academy of Sciences |
11:50-12:20 (GMT+8) | Multi-agent reinforcement learning from the perspective of model complexity | Feng Wu | University of Science and Technology of China |
Talks
Topic: Reinforcement Learning for Optimized Decision-making and Control of Intelligent Robots
Xin Xu, National University of Defense Technology
Time: 9:00-9:40 (GMT+8)
Abstract: This talk will analyze the technical requirements and research challenges of intelligent robots under complex environments. To deal with the above challenges, the major models and algorithm frameworks of reinforcement learning (RL) will be introduced, together with some recent advances of feature representation and receding-horizon policy optimization in reinforcement learning algorithms.Then, some applications of RL in autonomous control and human-machine cooperative driving of intelligent vehicles will be introduced.Finally, the future research directions in related areas will also be discussed.
Topic: Artificial intelligence methods in real-time fighting games
Dongbin Zhao, Chinese Academy of Sciences
Time: 9:40-10:20 (GMT+8)
Abstract: Real-time fighting game is a typical one-to-one character confrontation game, to win the opponent during the limited time by effectively hit, which is an important research direction in the field of game artificial intelligence (AI). In recent years, AI methods represented by deep reinforcement learning and statistical forward planning have made breakthroughs in games. This talk will briefly introduce the fighting game, together with the mainly used artificial intelligence methods, analyzes their advantages and disadvantages. I will focus on the proposed method combining statistical forward planning and enhanced opponent modeling with reinforcement learning (the champion of fighting game AI competition in 2020 Conference on Games), and draft some future research trends of related fields.
Topic: Reward Decomposition: Discover and Leverage Decomposable Structure in Deep Reinforcement Learning
Li Zhao, Microsoft Research Asia
Time: 10:20-10:50 (GMT+8)
Abstract: In many environments the reward can be decomposed into sub-rewards obtained from different sources. Such decomposition can be further leveraged to improve sample efficiency of DRL algorithms and/or to gain better interpretability. Most existing works on reward decomposition require prior knowledge(such as decomposed state) to learn decomposed reward. We propose novel reward decomposition methods that can decompose reward without prior knowledge as well as improve sample efficiency of DRL algorithms.
Topic: Path integral reinforcement learning and its application in control system
Xian Guo, Nankai University
Time: 10:50-11:20 (GMT+8)
Abstract: In recent years, reinforcement learning technology has made significant breakthroughs in many fields. However, reinforcement learning techniques based on Markov decision process often perform well only in discrete action situations, and are unstable in continuous control systems based on differential equations. This report introduces reinforcement learning algorithms based on path integral. The theoretical basis of the algorithms is Hamilton Jaccobi Bellman equation. For this equation, by introducing appropriate assumptions, the statistical solution is obtained. The solution of the differential equation is transformed into solving the mathematical expectation. Finally, the approximate estimation of the expectation and the current iterative optimal strategy are obtained. This report first introduces the basic principle of path integral reinforcement learning algorithms, and then introduces two specific applications of the method in the control system: (1) aiming at the problem of robot path tracking control, combined with the traditional nonlinear control algorithm, we propose a better intelligent path tracking method than the traditional control method; (2) for the attitude control problem of high-speed aircraft based on the constraints of attitude control problem and PID controller, an efficient, robust and safe attitude control algorithm is proposed. In view of these two specific control problems, we share the research ideas and put forward the research prospects of reinforcement learning in the field of control.
Topic: Model-based Reinforcement Learning
Junge Zhang, Chinese Academy of Sciences
Time: 11:20-11:50 (GMT+8)
Abstract: DeepMindās AlphaX (AlphaGo, AlphaZero, AlphaStar) have obtained great success in Go, Starcraft and all these systems consume huge computation resources. Few organizations can afford such huge cost to train an agent. Thus, data inefficiency becomes a major drawback for such advanced techniques. Model based reinforcement learning tries to capture the dynamics to greatly improve the data efficiency and is regarded as a promising framework to address such a limitation. This report will introduce the latest progress of model-based reinforcement learning and the three dilemmas faced by MBRL. Then we will discuss our work in this line of research. Finally, this report will summarize some possible research directions in the future.
Topic: Multi-agent reinforcement learning from the perspective of model complexity
Feng Wu, University of Science and Technology of China
Time: 11:50-12:20 (GMT+8)
Abstract: In recent years, multi-agent reinforcement learning has made a lot of important progress, but it still faces great challenges when applied to real problems. Part of this comes from the complexity of the reinforcement learning method itself, and the other part comes from the difficulty of multi-agent distributed decision-making. For example, the complexity of solving the MDP model of a single agent is P, while the complexity of the Dec-POMDP model of multiple agents is NEXP (much greater than P). The huge difference in model complexity determines that multi-agent distributed decision-making is fundamentally much more difficult than single-agent decision-making, and reinforcement learning as a learning-based solution method cannot be spared. At present, most enhanced algorithms have more or less implicit preconditions when applied to multi-agent decision-making. These conditions may weaken the complexity of the problem to a certain extent. When real problems do not meet these (implicit) conditions, these algorithms are usually difficult to perform satisfactorily. This report will discuss the design of multi-agent reinforcement learning algorithms from the perspective of model complexity, in the hope of benefiting the practical application of multi-agent reinforcement learning algorithms.
Live Link
DAI 2020: https://dai2020.163.com/m/#liveWorkshop Chairs
Contact Person
Dr. Chao Yu
Sun Yat-sen University, China
Email: yuchao3@mail.sysu.edu.cn
This page was generated by GitHub Pages using the Architect theme by Jason Long.